ALL PUBLICATIONS
the Best Undergraduate Design Rank: 2/632, 2020
The Exellcent Paper Presentation Award
The Exellcent Paper Presentation Award
Invited to expand into a journal
Conference Paper
C. Huang, Y. Yu and M. Qi, "Skin Lesion Segmentation Based on Deep Learning," 2020 IEEE 20th International Conference on Communication Technology (ICCT), Nanning, China, 2020, pp. 1360-1364. Link
C. Huang, A. Yu, Y. Wang and H. He, "Skin Lesion Segmentation Based on Mask R-CNN," 2020 International Conference on Virtual Reality and Visualization (ICVRV), Recife, Brazil, 2020, pp. 63-67. Link
T. Zhou and C. Huang, "UAV Automatic Docking Technology Based on Deep Learning," 2020 International Conference on Computing and Data Science (CDS), Stanford, CA, USA, 2020, pp. 448-453. Link
Y. Wang, Y. Rao, C. Huang, Y. Yang, Y. Huang and Q. He, "Using the Improved Mask R-cnn and Softer-nms for Target Segmentation of Remote Sensing Image," 2021 4th International Conference on Pattern Recognition and Artificial Intelligence (PRAI), Yibin, China, 2021, pp. 91-95. Link
Y. Yi, Y. Rao, C. Huang, S. Zeng, Y. Yang, Q. He and X. Chen, "Optimization of Quantum Key Distribution Parameters Based on Random Forest," 2021 4th International Conference on Pattern Recognition and Artificial Intelligence (PRAI), Yibin, China, 2021, pp. 164-168. Link
C. Huang, A. Yu and H. He, "Using combined Soft-NMS algorithm Method with Faster R-CNN model for Skin Lesion Detection," 2020 6th International Conference on Robotics and Artificial Intelligence (ICRAI), Nov 2020, pp. 5–8. Link
C. Huang, Y. Liu, J. Li, H. Tian and H. Chen, "Application of YOLOv5 for mask detection on IoT ," Proceedings of the 5th International Conference on Computing and Data Science, 2023. Link
Y. Lin, Q. Tang, H. Wang, C. Huang, E. Favour, X. Wang, X. Feng and Y. Yu, "Attention Enhanced Network with Semantic Inspector for Medical Image Report Generation," 2023 IEEE 35th International Conference on Tools with Artificial Intelligence (ICTAI), Atlanta, GA, USA, 2023, pp. 242-249. Link
C. Huang, J. Shen, B. Hu, M. Ali and J. Zhang, "Semantic and Visual Attention-Driven Multi-LSTM Network for Automated Clinical Report Generationn," 2024 The 38th Annual AAAI Conference on Artificial Intelligence (AAAI), Vancouver, Canada, 2024. PDF
J. Shen, M. Ali, C. Huang, B. Hu, X. Xie and J. Zhang, "Temporal Graph Neural Networks For Paper Recommendation on Dynamic Citation Networks," 2024 The 38th Annual AAAI Conference on Artificial Intelligence (AAAI), Workshop, Vancouver, Canada, 2024. PDF
Medical Imaging and Memristor
Medical Imaging
Remote Sensing
Remote Sensing
Quantum communication and machine learning
Medical Imaging
AIoT and Object Detection
Generative AI
Generative AI
Graph Neural Network and Recommendation Systems
The Cover Paper, 2023
The Best Paper and The Cover Paper, 2022, IEEE TIM
Journal Paper
C. Huang, Y. Liu, J. Li, H. Tian and H. Chen, "Application of YOLOv5 for mask detection on IoT ," Applied and Computational Engineering, Vol. 29, pp. 1-11, 2023. Link
Q. Lv, Y. Rao, S. Zeng, C. Huang and Z. Cheng, "Small-Scale Robust Digital Recognition of Meters Under Unstable and Complex Conditions," in IEEE Transactions on Instrumentation and Measurement, vol. 71, pp. 1-13, 2022. Link
Y. Rao, Q. Lv, S. Zeng, Y. Yi, C. Huang, Y. Gao, Z. Cheng and J. Sun, "COVID-19 CT ground-glass opacity segmentation based on attention mechanism threshold," in Biomedical Signal Processing and Control, vol. 81, 2023. Link
AIoT and Object Detection
Resource-Efficient AI and Object Detection
Medical Imaging
I am also a student reviewer
I am reviewing the paper “Multi-Channel Hypergraph Convolutional Network Guided by Hybrid Random Walks for Web API Recommendation, ” whose manuscript ID is IoT-34804-2024, now
Book
Participated in editing, the thesis (undergraduate project) was included in Chapter 7.
Y. Yu, X. Hu, Y. Fu, C. Huang, etc."Nanomemristors and Neuromorphic Computing (Neuromorphic Circuits for Nanoscale Devices)," 2022. Link
Memristive Neural Network, Nanocomputing and AI
Manuscript
C. Huang, Y. Liu, Z. Xia, S. Jiang, H. Tian, and Y. Yu, "GB-YOLO: a lightweight model for mask detection on IoT system," IEEE Internet of Things Journal, 2023. PDF (reviewing)
C. Huang, J. Shen, M. Ali, B. Hu, X. Xie, J. Zhang, X. Wang and Y. Yu, "AMI: Attention Threshold-based Fast Segmentation and Report Generation Network for Glaucoma," the 27th International Conference on medical image computing and computer-assisted intervention (MICCAI), 2024. Link
J. Zhang, Z. Zhang, L. Yeshi , Y. Yu, X. Wang, C. Huang, G. Luosang and N. Tashi, "Tibetan Medical Named Entity Recognition Based on Character-word-sentence Embedding Transformer," Conference Paper.
Abstract: Tibetan medical named entity recognition (Tibetan MNER) involves extracting specific types of medical entities from unstructured Tibetan medical texts. Tibetan MNER can provide important data support for the work related to Tibetan Medicine. However, existing Tibetan medical named entity recognition methods often fail to capture semantic features at varying levels of granularity and do not fully utilize the global information. This paper introduces an improved embedding representation, termed Character-words-sentence Embedding, aimed at enhancing the specificity and diversity of feature representations. By integrating the Character-word-sentence Embedding strategy into the Transformer, the model has the ability to leverage comprehensive semantic information, which can improve the performance of Tibetan medical named entity recognition. We evaluate our proposed model on datasets from various domains. The results show that the model effectively recognizes three types of entities in the Tibetan News dataset, achieving an F1 score of 93.20%, which represents an improvement of 0.84%. Additionally, the results on the Tibetan medical dataset, which we constructed, demonstrate its efficacy in recognizing five types of medical entities, with an F1 score of 70.96%, reflecting an increase of 0.91%.
K. Tan, D. Tashi, Z. Zhang, J. Zhang, L. Yeshi, C. Huang, Y. Yu, X. Wang, R. Dongrub, and N. Tashi, "Tibetan Handwritten Letter Recognition using Convolutional Neural Network," Conference Paper.
Abstract: This research presents an approach for Tibetan handwritten letter recognition employing Convolutional Neural Network. The proposed model demonstrates significant advancements in accurately recognizing Tibetan handwritten letters. The training phase achieved an accuracy of 98.97% and an loss rate of 3.51%, while the testing phase yielded a accuracy of 97.92%. The training accuracy of this model is higher than LeNet-5’s accuracy by 1.08%, and the training loss rate is lower by 13.41%. Additionally, individual testing of each letter was conducted to further assess the model's performance on a per-letter basis. The successful implementation of the CNN-based Tibetan handwritten letter recognition system showcases the potential for effective recognition of Tibetan handwritten characters, contributing to the broader field of letter recognition in diverse scripts.
J. Xu, C. Huang, Y. Yu, X. Wang, D. Tashi, R. Dongrub, N. Tashi, "Dynamic Convolutional Neural Network in Image Caption Task Based on Tibetan Images," Conference Paper.
Abstract: With the growing interest in computer vision and natural language processing,the task of generating descriptive captions for images has garnered significant attention.This paper explores the use of an improved CNN (Convolutional Neural Network )based in the task of generating captions for Tibetan images.In the field of image captioning, Tibetan is considered a low-resource language, characterized by a scarcity of available datasets. The key challenge lies in achieving better training outcomes with limited datasets. Additionally, in practical application scenarios, the relatively lower computational resources constrain the depth (number of convolutional layers) and width (number of channels) of Convolutional Neural Networks (CNNs), leading to a decrease in performance and ultimately limiting the expressive capabilities of the model.To address these challenges, this study adopts an experimental approach by introducing a network architecture based on dynamic convolutional kernels. The distinctive feature of this network lies in its ability to dynamically aggregate multiple parallel convolutional kernels through attention mechanisms. This approach offers advantages such as high computational efficiency and enhanced representational capacity, aiming to mitigate the limitations posed by the scarcity of datasets in the case of low-resource languages.Compared to traditional CNN networks, the dynamic convolutional neural network exhibits an average performance improvement of 1.1 BLEU score points on the COCO dataset. When applied to the COCO dataset with captions translated into Tibetan, the network shows an average improvement of 0.85 BLEU score points.
罗桑益西,洪涛,道吉扎西,张瑾,于永斌,王向向,黄成,范满平,洛桑嘎登,尼玛扎西, "面向《四部医典》典型藏医疾病的知识图谱构建," Journal Paper.
Abstract: 本文利用Neo4j图数据库构建藏医疾病知识图谱,实现了藏医知识的存储和可视化展示。本文从《藏医疾病库》收集数据,并将其整理成CSV格式的数据集。然后,利用py2neo库将这些数据导入Neo4j图数据库,成功构建了包含7139个实体节点、9300个实体关系的知识图谱,内容涵盖了疾病的病因、症状、治疗等多个方面。本文构建的藏医疾病知识图谱能直观地展示疾病与病因、症状、治疗之间的关系,并使用相似度计算以及拓扑链路预测算法进行数据分析,验证了图数据科学库对于藏医疾病知识图谱分析的有效性。这一研究不仅丰富了藏医知识的存储方式,还为未来对藏医的研究与应用奠定了坚实的基础。
M. Huo, Y. Li, C. Huang, Y. Yu, X. Wang, N. Tashi, D. Tashi and R. Dongrub, "Tibetan Handwritten Alphabet Recognition Based on Convolutional Neural Networks," Conference Paper.
Abstract: The Tibetan region has nurtured a wealth of culture and abundant natural resources. In this context, optical character recognition (OCR) plays a pivotal role in digitizing and protecting Tibetan documents. This paper proposes a Spiking Convolutional Neural Network model based on SNN (Spiking Neural Network) and CNN (Convolutional Neural Network). This research utilized a new dataset constructed using open-source datasets to train the model and evaluate the robustness and energy efficiency performance of this model. CNN networks have demonstrated significant potential in OCR field, and the integration of SNN networks brings robustness to traditional CNN networks while saving a considerable amount of energy during the training process. Experimental results indicate that, compared to traditional CNN model, the SCNN (Spiking Convolutional Neural Network) model not only effectively withstands various attacks, maintaining recognition accuracy, but also significantly optimizes the computational power required during training. This work represents a significant breakthrough for deploying Tibetan text recognition software on mobile edge devices.
C. Huang, D. Zhaxi, T. Sheng, B. Chen,Y. Yu, X.g Wang and N. Tashi, "Tibetan Data Augmentation: Applications of Handwriting Imitation with GANs," Conference Paper.
Abstract: As awareness increases regarding the preservation of traditional handwritten documents from the Chinese-Tibetan ethnic group, the lack of labeled Tibetan handwriting data impedes progress in fields such as computer vision and other commercial applications. This paper proposes a data augmentation approach using handwriting imitation GANs for Tibetan handwriting generation, which generates handwritten images accompanied by corresponding Tibetan textual labels. In addition, this study uniquely constructs a dataset featuring the Khyug-yig style of Tibetan calligraphy, thereby improving the diversity of Tibetan handwriting documents. The experiments involve Tibetan handwriting datasets, including numerals in the Umê style, consonants in the Uchen style, and words in the Khyug-yig style of Tibetan calligraphy. Consequently, the model imitated recognizable Tibetan numeral and consonant handwriting, achieving Fréchet Inception Distance (FID) scores of 14.45 and 27.63, respectively. Its attempts to imitate Khyug-yig Tibetan words, known for their complex structures, resulted in a test FID of 32.37. These results demonstrated the feasibility of utilizing generated handwriting images for Tibetan data augmentation through our method.
Z. Zhang, Y. Yu, X. Wang, H. Xia, J. Xu, J. Xu, N. Tashi and C. Huang, "Chinese-Tibetan Machine Translation Model based on Deep Neural Network," Conference Paper.
Abstract: In the field of natural language processing, Chinese-Tibetan machine translation faces unique challenges. These challenges arise from significant differences in grammatical structures, vocabulary usage, and expression habits between the two languages, as well as the lack of sufficient bilingual corpora. Additionally, the complex script system of the Tibetan language and the scarcity of digital resources further increase the difficulty of translation, which are pressing issues that need to be addressed. In response to these requirements, this paper has incorporated cutting-edge deep neural network architectures for the training of Chinese-Tibetan neural machine translation models. This paper has trained a Chinese-Tibetan neural machine translation model based on the Transformer structure and has also trained another model using a Lightweight Convolutional structure for performance comparison. The models in the article were evaluated using the BLEU-4 metric, and the results were convincing. In the Chinese-Tibetan translation task, the Transformer model demonstrated good performance, with a BLEU score of 22.24, indicating the effectiveness of the Transformer in handling Tibetan linguistic structures. In comparison, the lightweight convolutional model reached 21.67. While having similar translation accuracy, it significantly reduced training time and improved training efficiency, which is also a key factor for large-scale NMT tasks. Furthermore, this paper, by comparing different tokenization and Byte Pair Encoder (BPE) methods, found that appropriate data preprocessing plays a key role in improving the translation accuracy of low-resource languages.
C. Huang, J. Xu, Y. Yu, X. Wang, Z. Zhang, T. Tsering and N. Tashi, "Accelerating Tibetan-Chinese Machine Translation: Leveraging FNet for Efficient Language Processing," Conference Paper.
Abstract: This paper introduces a novel approach to Tibetan-Chinese machine translation by applying the FNet model, originally proposed for Natural Language Processing tasks. FNet, which substitutes the self-attention mechanism in Transformers with Fourier transforms, offers computational efficiency and speed. We adapt FNet for the specific linguistic features of Tibetan and Chinese, handling their unique syntactic and semantic challenges. Our approach includes data collection, preprocessing, and training the FNet model on a parallel Tibetan-Chinese corpus. We evaluate the model's translation accuracy and efficiency, comparing it with traditional Transformer-based models. The results demonstrate that FNet, while maintaining comparable accuracy, significantly enhances translation speed and reduces computational requirements, making it an effective tool for Tibetan-Chinese translation tasks. This study paves the way for efficient language translation models in less-resourced language pairs, emphasizing the balance between computational efficiency and translation quality.
AIoT and Edge Computing
Generative AI and LLM
Some Interesting Work
Y. Sun, J. Qi, R. Wen, H. Tian, C. Huang and K. Zao, "Pedestrian Tracking based on Improved YOLOv5," PDF.
R. Wen, J. Qi, Y. Sun, H. Tian, C. Huang and K. Zao, "Faced-mask Detection based on YOLOv4," PDF.
H. Tian, J. Qi, Y. Sun, Z. Zhang, R. Wen, C. Huang and K. Zao, "Auxiliary Use of YOLOv5 in FPS Shooting Competitive Games," PDF.
LoR Recommender
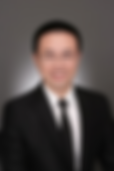
Ph.D. (UESTC)
Associate Professor, UESTC
Instructor, Advisor, Graduate Advisor
AI, EDA, Memristor
Integrated Circuit
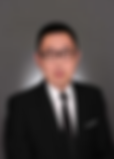
Ph.D. (UESTC)
Associate Professor, UESTC
Instructor, Advisor
AI, Cyber Security
AR, VR, Quantum computing
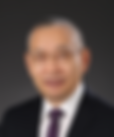
Ph.D. (THU)
Professor, THU
Advisor
IEEE Fellow
Chip design, EDA
AI chip acceleration
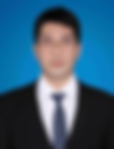
Ph.D. (UCSD)
Associate Professor, NPU
Advisor, Tutor
Chip design, EDA
AI chip acceleration
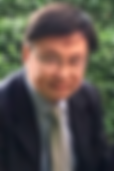
Ph.D. (Harvard)
Professor, CUHK
Advisor, Tutor
SMIEEE
IoT Expert, Trusted Edge Computing and Analytics